Cost-Saving Strategies: Predictive Analytics for Healthcare Staffing
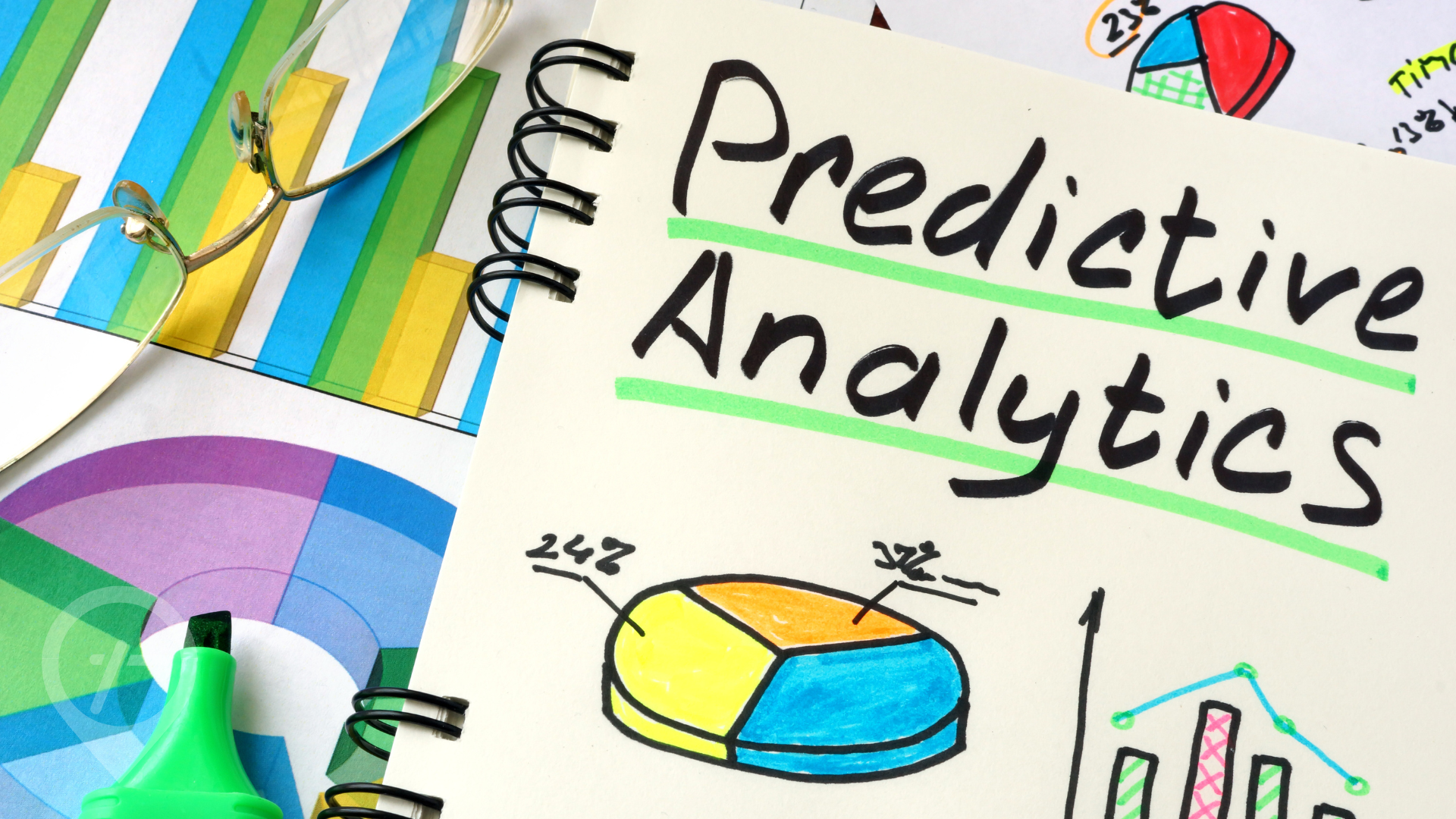
Healthcare organizations face significant challenges in managing staffing levels and controlling overtime costs. These challenges can lead to financial strain, decreased employee morale, and compromised patient care. Predictive analytics healthcare offers a solution by providing data-driven insights that help optimize staffing levels and minimize overtime. This article explores the impact of overtime costs, the role of predictive analytics solutions in workforce management, and strategies for implementing data-driven scheduling to improve staffing efficiency and reduce expenses.
Understanding the Impact of Overtime Costs
In healthcare settings, overtime costs carry significant implications beyond their financial impact. Excessive overtime can have several negative consequences:
Employee Morale: Prolonged periods of overtime can lead to burnout, job dissatisfaction, and higher turnover rates among healthcare staff. Employees working extended hours often experience fatigue and stress, which can diminish their overall job satisfaction and productivity. This, in turn, affects the workplace environment, leading to higher turnover rates and increased recruitment and training costs.
Patient Care: Fatigued employees are more prone to errors, which can affect the quality of patient care and safety. Studies have shown that overworked healthcare professionals are more likely to make mistakes in patient assessments, medication administration, and other critical tasks. These errors can compromise patient safety, lead to adverse outcomes, and increase the risk of medical malpractice claims.
Financial Impact: High overtime costs increase operational expenses, affecting the organization's bottom line. In addition to the direct costs of paying overtime wages, there are indirect costs associated with decreased productivity, higher absenteeism, and increased staff turnover. These financial burdens can strain the organization's resources and limit its ability to invest in other critical areas, such as technology, training, and patient care initiatives.
The Role of Predictive Analytics in Workforce Management
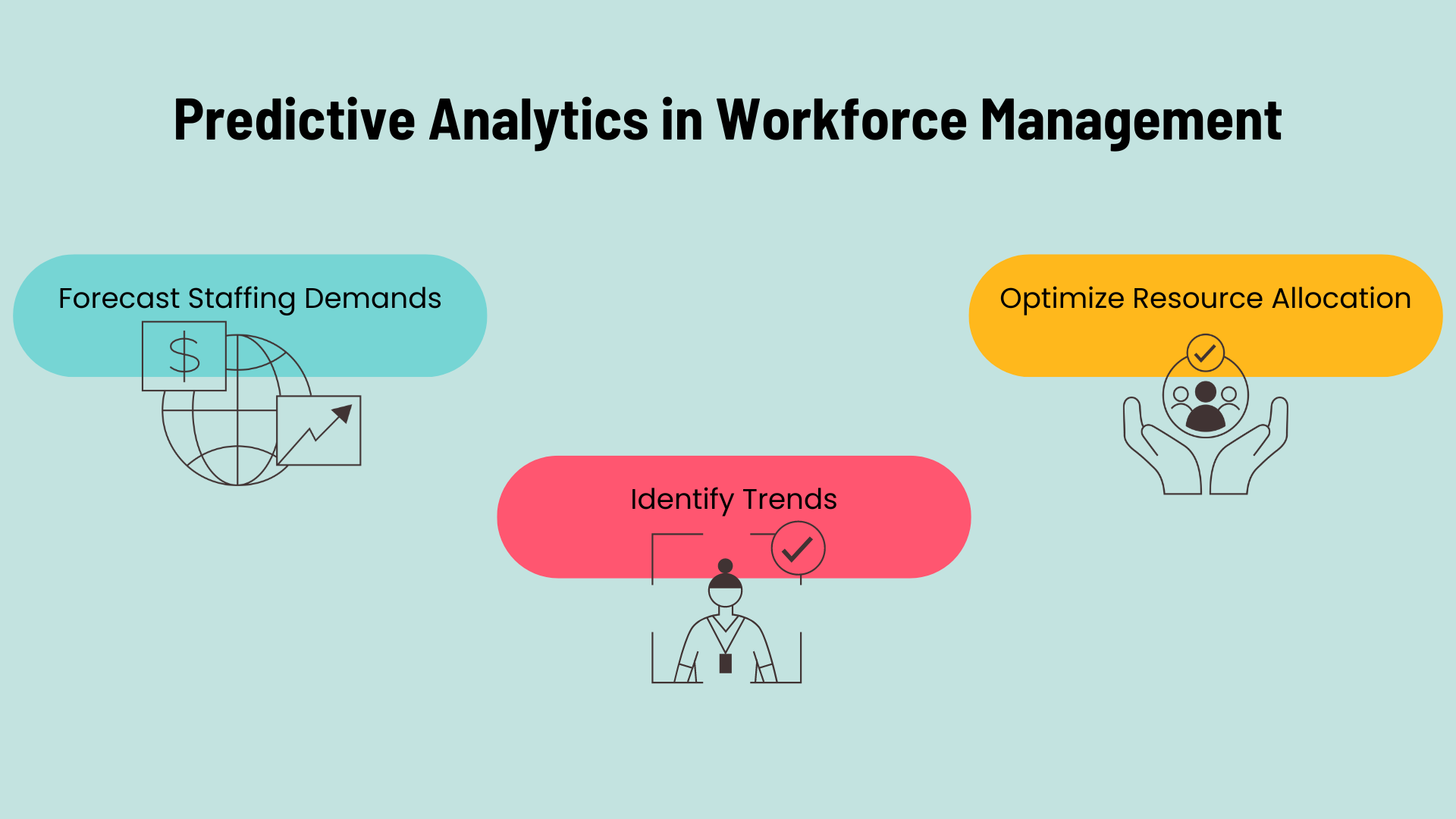
Predictive analytics healthcare involves using data, statistical algorithms, and machine learning techniques to identify the likelihood of future outcomes based on historical data. In healthcare staffing, predictive analytics tools can:
Forecast Staffing Demands: Predictive analytics solutions analyze historical data to forecast future staffing needs, helping organizations plan more effectively. These models consider various factors, such as patient admission rates, seasonal trends, and staff availability, to provide accurate predictions of staffing requirements. This enables healthcare organizations to anticipate demand and allocate resources accordingly.
Identify Trends: By recognizing patterns and trends in staffing and patient volume, healthcare organizations can make informed decisions to optimize workforce management. Predictive analytics software healthcare can uncover insights into factors that influence staffing needs, such as peak admission times, patient demographics, and seasonal illnesses. These allow organizations to develop targeted strategies to address staffing challenges and improve operational efficiency.
Optimize Resource Allocation: Predictive analytics tools healthcare can help allocate resources more efficiently, ensuring that the right number of staff is available at the right time. By aligning staffing levels with patient care demands, organizations can reduce the risk of understaffing or overstaffing, minimize overtime, and enhance the overall quality of care.
4 Steps to Overcoming Overtime Costs with Healthcare Data Analytics
1. Identify Overtime Patterns with Data Analysis
Predictive analytics solutions can analyze historical data to identify patterns and factors contributing to overtime usage. Common factors include:
Seasonal Fluctuations: Patient volume often varies with seasons, leading to fluctuating staffing needs. For example, influenza season typically sees a surge in hospital admissions, while elective procedures may increase during certain months. Understanding these seasonal patterns allows organizations to plan staffing levels accordingly and mitigate the impact of fluctuating patient volumes.
Patient Volume: Sudden increases in patient admissions or emergency cases can result in unplanned overtime. Predictive analytics algorithms healthcare can help identify trends in patient volume, such as the impact of local events, holidays, or public health crises. By anticipating these spikes, healthcare organizations can adjust staffing levels to ensure adequate coverage without relying on overtime.
Staff Scheduling Preferences: Employees' preferences and availability can impact scheduling and overtime. For instance, some staff members may prefer certain shifts or have limitations on their availability. Predictive analytics software for healthcare providers can incorporate these preferences into scheduling models, balancing employee needs with organizational requirements to create optimal schedules that minimize overtime.
2. Forecast Staffing Needs to Minimize Overtime
Predictive models can forecast future staffing needs by considering historical data and other factors, such as upcoming events or seasonal trends. Accurate forecasting helps prevent:
Understaffing: Insufficient staffing can lead to overworked employees and increased overtime. Predictive analytics in healthcare industry trends can identify periods of high demand, allowing organizations to schedule additional staff or arrange for temporary support to prevent understaffing. This ensures that patient care needs are met without overburdening existing staff.
Overstaffing: Excessive staffing can result in unnecessary labor costs and reduced efficiency. Predictive models can highlight periods of lower demand, enabling organizations to adjust staffing levels and avoid overstaffing. This approach optimizes labor costs while maintaining adequate coverage for patient care.
3. Implement Data-Driven Scheduling Strategies
To minimize overtime, healthcare organizations can adopt various scheduling strategies informed by predictive analytics:
Flexible Scheduling: Adjusting shift schedules based on predicted patient volume and staff availability. Flexible scheduling allows organizations to match staffing levels with anticipated demand, reducing the likelihood of overtime. This approach can include staggered shift start times, variable shift lengths, and part-time or per diem staffing options.
Shift Adjustments: Modifying shift lengths or start times to better align with demand. Predictive analytics can identify peak periods of patient activity, enabling organizations to adjust shift schedules accordingly. For example, extending shifts during high-demand periods or reducing shift lengths during slower times can help balance staffing levels.
Predictive Scheduling: Using predictive models to create schedules that optimize staffing levels and reduce the likelihood of overtime. Predictive scheduling incorporates historical data, employee preferences, and anticipated demand to generate efficient schedules that meet organizational needs while minimizing the risk of overtime. This approach ensures that staffing levels are dynamically adjusted based on real-time insights.
4. Monitor and Adjust Strategies for Continuous Improvement
Continuous monitoring and evaluation of staffing practices are essential for identifying areas for improvement. Predictive analytics facilitates real-time monitoring of staffing levels and overtime usage, allowing organizations to:
Identify Trends: Detect emerging patterns and trends in staffing and overtime. Continuous analysis of staffing data can reveal insights into factors that influence overtime, such as changes in patient volume, staff availability, and scheduling practices. These insights enable organizations to adjust their strategies proactively to address potential issues.
Make Adjustments: Implement changes based on data-driven insights to optimize workforce allocation. Real-time monitoring allows organizations to respond quickly to fluctuations in demand, adjusting staffing levels and schedules as needed to maintain optimal coverage. This approach minimizes overtime and ensures that patient care needs are consistently met.
Examples of adjustments include reallocating staff based on predicted patient volume or modifying shift schedules to better match demand. For instance, if predictive analytics indicates an upcoming surge in patient admissions, organizations can schedule additional staff in advance to prevent overtime. Similarly, if data shows a decline in patient volume, organizations can reduce staffing levels to avoid unnecessary labor costs.
Conclusion
Healthcare organizations can leverage predictive analytics solutions to optimize staffing levels and minimize overtime costs. By understanding the impact of overtime, utilizing predictive analytics, and implementing data-driven scheduling strategies, organizations can improve operational efficiency, enhance employee satisfaction, and ensure high-quality patient care.